Big Data & Analytics
Unleash the power of big data, fuel effective data-driven analytics.
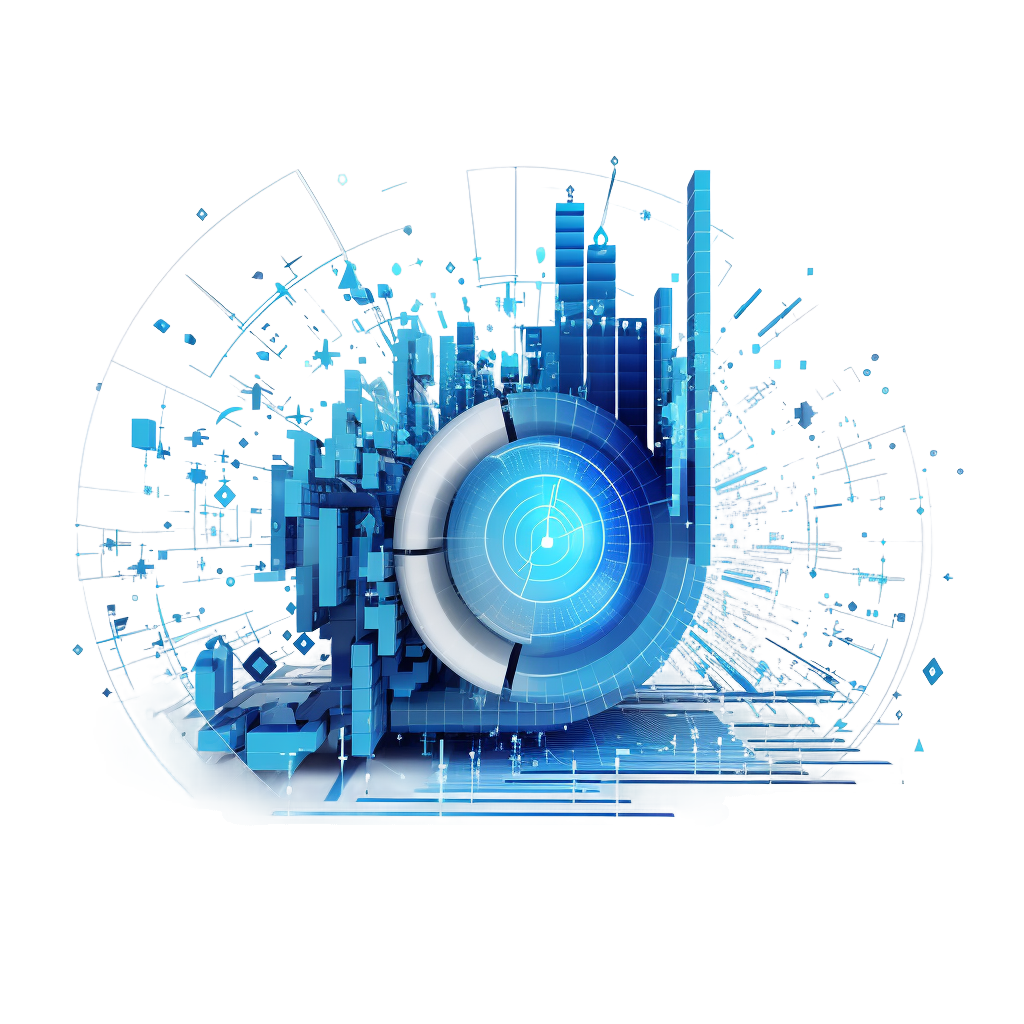
A daily tidal wave of data is constantly reshaping our world. Unlocking the strategic insights within this deluge, through the power of big data and analytics, can transform organizations, turning their raw information into a competitive advantage. However, this data-rich environment demands expertise covering data-centric technical skills, as well as business acumen.
As a big data and analytics consulting firm, Dataiso helps you completely get the most out of your data to achieve data-driven success. We provide tailored (big) data analytics solutions that transform your complex data into actionable intelligence, fueling innovation and growth across your organization.
Your challenges
Your challenges
Big data is defined by its volume, velocity, variety, value, and veracity (the 5 V’s), representing extremely large datasets that are constantly evolving. This growth creates quintillions of bytes daily, encompassing structured, semi-structured, and unstructured information.
Traditional data management struggles with the sheer volume and complexity of modern datasets. As a result, this situation has driven the adoption of a modern data-at-scale approach called “big data analytics”, which enables efficient storage, processing, and insight extraction from massive datasets in motion—as well as those at rest. However, many organizations are still determining the most effective approach for their big data and/or analytics projects.
Dataiso has identified key challenges limiting organizations’ ability to harness the full power of big data and analytics.
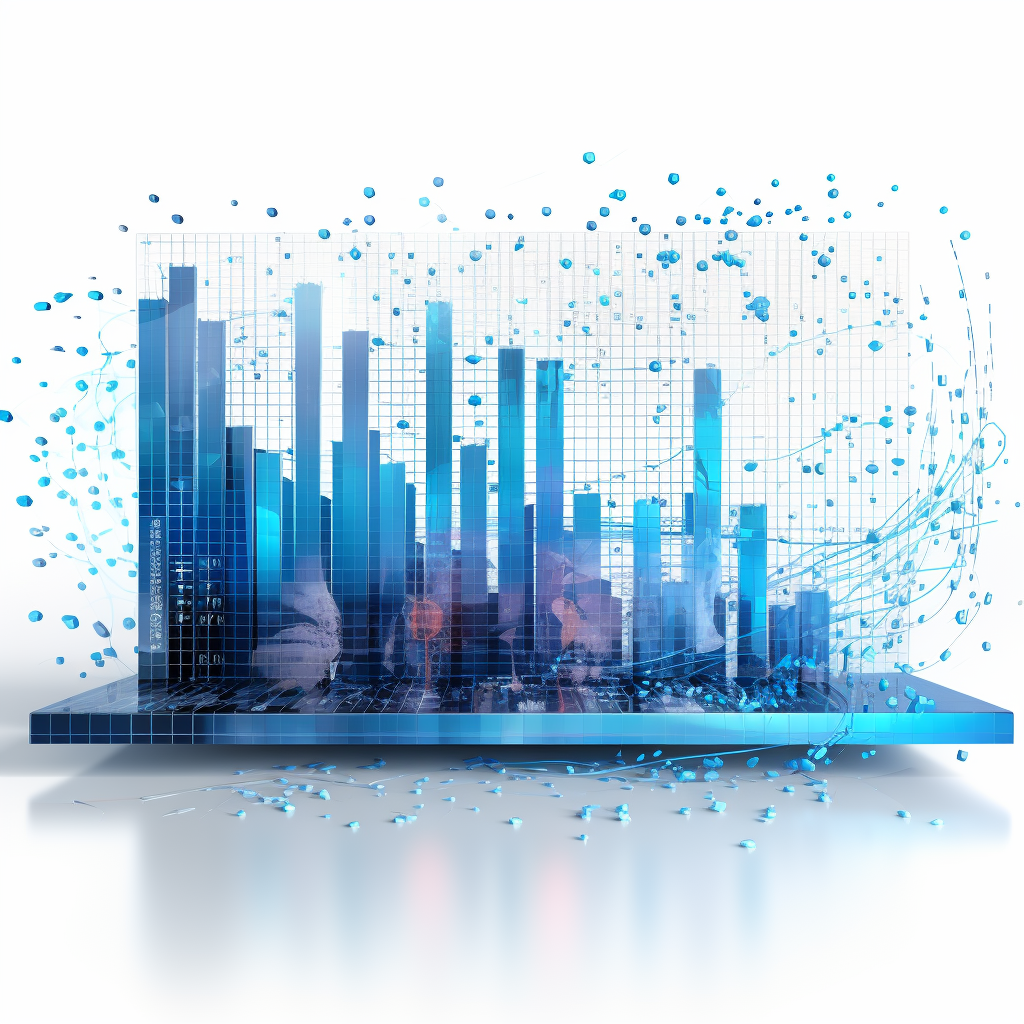
Fragile big data adoption
Big data's complexity hinders adoption, leaving organizations reliant on outdated technologies. This results in costly hardware investments instead of modern big data and analytics architectures.
High maintenance costs
Traditional data platforms fail to manage the high volume and variety of modern data, hindering optimal data management. This incurs costly maintenance for adequate performance.
Uncontrolled data explosion
Scaling big data and analytics environments proves challenging. Many organizations struggle with a huge variety of data sources, making data management, including data analysis, harder.
Unreliable data and security risks
Evolving business requirements expose flaws in unreliable data collection, jeopardizing data integrity. Consequently, organizations prioritize storage and analysis over data security.
Skills gap in big data and analytics deployment
Talent shortages drive unchecked big data and analytics deployments across organizations. This creates disconnected data silos and conflicting analytics that hinder decision-making
Our key factors of success
Our key factors of success
Unlocking the power of big data requires a strategic approach and advanced analytical techniques. The key factors prioritized by Dataiso help deliver meaningful insights from your large and complex datasets.
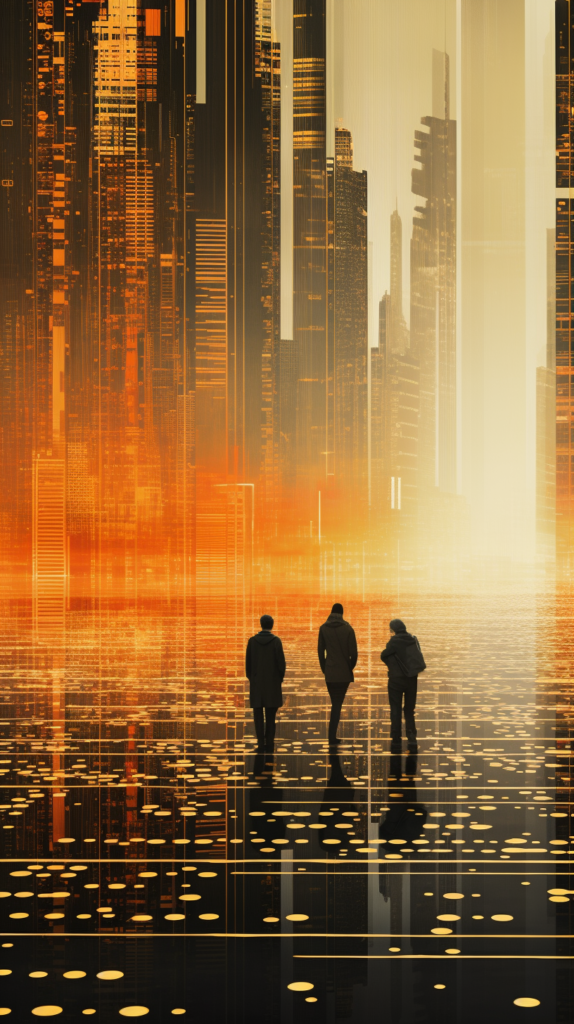
Organizations unfamiliar with big data and analytics often resist such initiatives. Successfully implementing them requires first gaining top management buy-in to overcome employee resistance.
Since every organization has unique needs, and data sets, selecting the right big data technology is critical. The vast volume and variety of data require different approaches.
Integrating big data and analytics with business processes fosters big data literacy and user participation. This leads to valuable feedback and more relevant solutions.
High-quality data is crucial for building user trust; unreliable data won’t be used for decision-making. Robust data security is equally vital to protect against financial and reputational risks, ensuring the organization’s stability.
Big data and analytics speed requirements vary by use case. Real-time queries demand fast load times and responsiveness, while background tasks tolerate slower processing. Enabling scalability optimizes speed and reduces infrastructure costs.
Integrating big data and analytics with DevOps practices streamlines deployment. CI/CD (Continuous Integration/Continuous Delivery) automates operations across storage, ingestion, analysis, and dissemination, accelerating the big data and analytics lifecycle. Orchestration tools, meanwhile, centralize processing management, enhancing oversight.
Slow processing of a massive volume of data can impact user experience and cripple operational and business activities. Proactively deploying suitable methods, resources, and tools is important to ensure optimal performance levels.
Our approach
Our approach
At Dataiso, we don’t just process your data; we solve your problems. We rely on an approach that helps you deliver actionable insights that drive innovation and strategic advantage by leveraging the power of big data and analytics through a tailored People-Process-Technology (PPT) framework.
In brief, we partner with your data team to define clear objectives and key performance indicators (KPIs), using hybrid strategies to iteratively develop and test custom big data and analytics solutions.
Our services
Our services
Dataiso provides cutting-edge big data and analytics services to help organizations achieve real-world results. We go beyond theoretical methods, delivering bespoke solutions that address your specific challenges and unlock new opportunities.
Big data and analytics strategy and roadmap
- Maximize return on investment (ROI) by aligning big data and analytics objectives with the overall strategy.
- Drive growth by identifying high-impact opportunities where big data and analytics can make a significant difference.
- Create a comprehensive big data and analytics roadmap for successful implementation strategies.
- Define the appropriate big data and analytics technologies and tools to meet unique business needs and drive innovation goals.
Strengthen big data and analytics scaling strategies by implementing big data and analytics operations (BDOps/AnalyticsOps) principles.
- Demonstrate the value of big data and analytics through compelling proofs of concept (PoCs) and proofs of value (PoVs).
Big data and analytics audit and diagnosis
- Assess all existing big data and analytics practices, policies, and technologies.
- Identify gaps between the organization’s current state and big data and analytics best practices, including both technical and functional discrepancies.
- Assess big data and analytics health and observability, including models, pipelines, quality, consistency, and accessibility.
- Evaluate big data and analytics systems’ strengths and weaknesses using methods like performance testing, and user feedback.
- Review big data and analytics ethics, sustainability, security, privacy, and compliance.
- Benchmark big data and analytics maturity against industry standards with proven maturity models.
- Maximize big data and analytics investments through efficient optimization plans.
Big data and analytics architecture deployment
- Implement tailored big data and analytics architectures, such as Lambda, Kappa, and Data Lake, for specific needs.
- Integrate best-in-class big data and analytics components, languages, and tools.
- Ensure seamless big data and analytics deployment on cloud platforms, on-premises infrastructure, or hybrid environments.
- Optimize big data and analytics infrastructure through smarter performance tuning techniques and efficient resource allocation.
- Strengthen big data and analytics security and governance through proactive measures leveraging data protection and privacy best practices.
- Streamline and scale deployments with robust big data and analytics operations (BDOps/AnalyticsOps) practices.
Data storage layer design
- Build a robust and scalable data storage layer aligned with specific needs.
- Integrate best-of-breed data storage technologies like data lakes, data warehouses, and data marts.
- Enforce data retention and archival policies for safety and compliance.
- Reduce storage costs while ensuring data quality with efficient data storage techniques.
- Optimize data accessibility, reliability, and performance using best practices
- Take control of data storage versioning and change management through effective version control systems.
- Enable ongoing data storage refinement and validation with regular reviews and updates.
Data ingestion pipelines development
- Build efficient data pipelines for batch, stream, and event-driven data ingestion.
- Enable various use cases by integrating data from diverse sources such as structured, unstructured, and semi-structured datasets effectively.
- Design smooth data validation and cleansing processes.
- Maintain high data quality and consistency throughout the ingestion process
- Boost data ingestion performance and scalability using efficient ETL (Extract, Transform, Load) and ELT (Extract, Load, Transform) methods.
- Facilitate data ingestion accuracy, completeness, integrity, and consistency with efficient data ingestion pipeline versioning and change control protocols.
- Enable ongoing data ingestion refinement and validation through regular reviews and updates.
Data processing
- Apply advanced data processing techniques based on specific use cases: batch, stream, and event-driven processing.
- Cleanse, transform, and enrich data for effective data analytics and more.
- Create seamless data preparation pipelines for efficient data processing.
- Implement robust data quality checks and validation for large-scale datasets.
- Ensure data processing performance and scalability for more effective real-time analytics..
- Elevate data processing accuracy, completeness, integrity, and consistency with efficient data processing pipeline versioning and change control protocols.
- Enable ongoing data processing refinement and validation through regular reviews and updates.
Data analysis and visualization
- Uncover hidden patterns and trends with advanced methods based on exploratory data analysis (EDA) and data mining.
- Boost problem-solving capabilities using precise diagnostic analysis.
- Forecast future trends and outcomes by applying predictive analysis through machine learning (ML) techniques.
- Improve decision-making through prescriptive analysis.
- Empower employees with an easy understanding of complex data through AI-augmented interactive dashboards and reports.
- Gain a competitive advantage with analytics-driven innovation using advanced and augmented analytics fueled by data intelligence.
- Strengthen accuracy, completeness, integrity, and consistency with efficient data analysis models, dashboard versioning, and change control protocols.
- Enable ongoing model and dashboard refinement and validation through regular reviews and updates.
Data orchestration
- Unify data ecosystems by integrating disparate data sources.
- Ensure seamless data ingestion and processing with automated data pipelines.
- Optimize resource utilisation and cost-efficiency through intelligent workload management.
- Facilitate decision-making with real-time data availability and accuracy.
- Ensure data accuracy, completeness, integrity, and consistency with efficient data orchestration mechanism versioning and change management protocols.
- Enable ongoing data orchestration refinement and validation through regular reviews and updates.
Big data and analytics migration
- Assess big data and analytics migration requirements for cloud or on-premises, including gap and risk analysis.
- Validate existing big data and analytics compatibility, scalability, and performance through rigorous benchmarking and stress-testing methods.
- Build robust big data and analytics migration plans addressing technical, operational, and business specifications.
- Implement comprehensive cutover and rollback plans, leveraging robust testing and validation methods.
- Seamlessly migrate big data and analytics assets to the target platform, with minimal disruption and risks.
- Support enhanced features and patches for better security and reliability with upgraded platform versions.
- Validate (big) data integrity and quality post-migration, ensuring accuracy, completeness, and consistency of business-critical information.
Big data and analytics security and governance
- Safeguard big data and analytics landscape with efficient security measures (e.g., data classification, encryption, access controls) following industry standards, including ISO 27001.
- Establish transparency, accountability, and compliance with regulations (e.g., Data Act, GDPR, CCPA) through future-proof big data and analytics governance.
- Strengthen data confidentiality, integrity, and availability using a comprehensive CIA triad model aligned with industry standards like ISO 8000 and ISO 25012.
- Uphold fairness, explainability, and privacy by addressing AI ethics and bias throughout the big data and analytics lifecycle.
- Enhance big data and analytics monitoring and preventive methods through proactive data observability.
- Integrate big data and analytics governance with overall data governance frameworks and best practices, including comprehensive policies and procedures.
Your benefits
Your benefits
- Actionable data-driven insights and opportunities.
- Optimized business outcomes and predictions.
- High-impact data analytics.
The potential of big data and analytics is vast, as data volumes and technologies keep evolving. Investing in big data and analytics implies to deal with a challenging landscape of infrastructure and methodologies.
Get in touch to explore how Dataiso can help you achieve long-lasting success in big data and analytics.